How Yann LeCun Deep Learning Revolutionized Modern AI
Estimated reading time: 7 minutes
Yann LeCun is a name synonymous with the revolution in artificial intelligence (AI) and has profoundly shaped the landscape of modern AI through his pioneering work in deep learning. His contributions, particularly in the development of Convolutional Neural Networks (CNNs), have not only transformed computer vision but have also paved the way for many applications that we rely on today.
This blog post delves into Yann LeCun’s groundbreaking work and explores how deep learning has revolutionized the field of Artificial Intelligence (AI), making tasks once considered impossible now achievable
Who is Yann LeCun: A Pioneer in Deep Learning
Yann LeCun was born in 1960 and is a French-American computer scientist renowned for his work in machine learning, computer vision, mobile robotics, and computational neuroscience. He earned his Ph.D. in Computer Science from Sorbonne University in 1987 and later joined AT&T Bell Labs, where he began his groundbreaking work on CNNs.
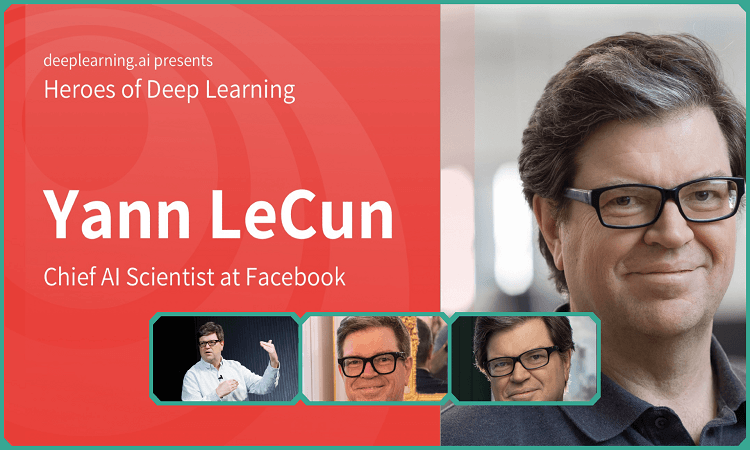
Currently, he holds the position of Silver Professor at NYU’s Courant Institute and serves as the Chief AI Scientist at Meta. Yann LeCun’s contributions to the field have earned him numerous accolades, including the prestigious Turing Award in 2018, which he shared with Yoshua Bengio and Geoffrey Hinton, solidifying their title as the “Godfathers of AI”.
The Foundation of Convolutional Neural Networks (CNNs)
Yann LeCun’s most influential contribution to AI is arguably the development of Convolutional Neural Networks (CNNs). He introduced CNNs in 1988, a type of deep neural network designed to process data with a grid-like topology, such as images.
Convolutional Neural Networks (CNNs) use convolutional layers that apply filters to the input data, enabling the network to automatically learn spatial hierarchies of features. This architecture has proven highly effective for various applications, including:
- Image Classification: Identifying objects within images
- Object Detection: Locating and classifying multiple objects in a single image
- Facial Recognition: Enhancing security systems and social media applications
The success of CNNs lies in their ability to automatically learn features from raw data, reducing the need for manual feature extraction. This innovation has led to significant advancements in fields such as autonomous driving, medical imaging, and augmented reality.
Advancements Beyond CNNs
Yann LeCun’s influence extends beyond CNNs. He has also been instrumental in the development of various deep learning techniques that have shaped the landscape of artificial intelligence. Some notable advancements include:
- Transfer Learning: Leveraging pre-trained models to improve performance on new tasks with limited data
- Generative Adversarial Networks (GANs): Exploring the potential of GANs in generating realistic data
These advancements have broadened the scope of deep learning applications and further solidified Yann LeCun’s position as a leading figure in the field.
The Impact of Yann LeCun’s Work
Yann LeCun’s impact on deep learning is profound. His research has not only advanced the field but has also inspired a new generation of AI researchers.
The integration of deep learning into everyday applications has revolutionized industries, making tasks that were once considered impossible achievable. His work has laid the groundwork for the AI revolution we are witnessing today
The Rise of Deep Learning in Modern AI
Deep learning, fueled by Yann LeCun’s innovations, has become a dominant force in modern AI. Its ability to automatically learn complex patterns from vast amounts of data has enabled breakthroughs in various domains.
Here are some key areas where deep learning has made a significant impact:
- Computer Vision: Deep learning has revolutionized computer vision, enabling machines to “see” and interpret images with remarkable accuracy. CNNs have become the standard architecture for image recognition, object detection, and image segmentation tasks. This has led to applications such as self-driving cars, facial recognition systems, and medical image analysis.
- Natural Language Processing (NLP): Deep learning has also transformed NLP, enabling machines to understand and generate human language. Recurrent Neural Networks (RNNs) and Transformers have become the go-to architectures for tasks such as machine translation, text summarization, and sentiment analysis. This has led to applications such as chatbots, virtual assistants, and language translation services.
- Speech Recognition: Deep learning has significantly improved speech recognition accuracy, enabling machines to transcribe spoken language with near-human precision. This has led to applications such as voice-controlled devices, transcription services, and voice search.
- Robotics: Deep learning is playing an increasingly important role in robotics, enabling robots to perceive their environment and make autonomous decisions. This has led to applications such as self-driving cars, industrial automation, and healthcare robots.
Current Trends and Future Directions
The field of deep learning is constantly evolving, with new trends and developments emerging at a rapid pace. Some of the most promising areas of research include:
- Ethical AI: With the increasing deployment of AI systems, there is a growing concern about ethical considerations such as bias, fairness, and transparency5. Researchers are working on developing techniques to mitigate bias in AI models and ensure that they are used responsibly.
- Human-Centric AI: There is a growing focus on designing AI systems that are aligned with human values and needs. This involves developing AI systems that are transparent, explainable, and accountable.
- Generative AI: Generative AI models, such as GANs and Variational Autoencoders (VAEs), are capable of generating new data that resembles the training data. This has led to applications such as image synthesis, music generation, and text generation.
- Quantum Computing: Quantum computing has the potential to revolutionize deep learning by enabling the training of more complex models and the processing of larger datasets. Quantum machine learning is an emerging field that explores the intersection of quantum computing and machine learning.
- AI in the Workplace: AI is being integrated into the workplace to enhance productivity and creativity. AI-powered tools can automate tasks, provide insights, and augment human capabilities.
FAQs
What is Deep Learning?
Deep learning is a subfield of machine learning that uses artificial neural networks with multiple layers to analyze data and make predictions. These networks are designed to automatically learn and extract complex features from raw data, enabling them to perform tasks such as image recognition, natural language processing, and speech recognition with high accuracy.
How has Deep Learning changed Artificial Intelligence?
Deep learning has revolutionized AI by enabling machines to perform tasks that were previously considered impossible. Its ability to automatically learn complex patterns from vast amounts of data has led to breakthroughs in various domains, including computer vision, natural language processing, and robotics.
What are Convolutional Neural Networks (CNNs)?
Convolutional Neural Networks (CNNs) are a type of deep neural network specifically designed for processing data with a grid-like topology, such as images. They use convolutional layers that apply filters to the input data, enabling the network to automatically learn spatial hierarchies of features. CNNs have become the standard architecture for image recognition, object detection, and image segmentation tasks.
What is Transfer Learning and why is it important?
Transfer learning is a technique in machine learning where a model trained on one task is reused as the starting point for a model on a second task. It is particularly useful when there is limited data available for the second task, as the pre-trained model can leverage the knowledge gained from the first task to improve performance.
What are the ethical considerations surrounding Deep Learning?
As deep learning systems are increasingly deployed in various applications, ethical considerations such as bias, fairness, and transparency become paramount. It is crucial to ensure that these systems are free from bias and that they are used responsibly and ethically.
In Conclusion
Yann LeCun’s pioneering work in deep learning, particularly his development of Convolutional Neural Networks (CNNs), has revolutionized modern AI. His contributions have not only transformed computer vision but have also paved the way for numerous applications that we rely on today.
Deep learning has become a dominant force in AI, enabling breakthroughs in various domains. As the field continues to evolve, it is crucial to address ethical considerations and ensure that AI systems are developed and used responsibly. For those interested in delving deeper into this fascinating field, exploring resources like NVIDIA’s Deep Learning Resources is a great starting point
- The Truth About Home Security Systems Everyone Should Know - February 15, 2025
- Best Weight Loss Food Programs: 18 Tips and Tricks for Success - February 15, 2025
- Is Your Google Business Profile Safe? Find Out Now to Fix It - February 15, 2025
Discover more from Akinpedian
Subscribe to get the latest posts sent to your email.